Designing a Framework on How LLMs could be tuned to not be biased against Gender
- ProBonoLegalBlogs
- Jul 9, 2024
- 10 min read
The Author of this Blog Article is Ms. Simar Aneja, a second year law student pursuing B.A.LL. B, from National University Of Juridical Sciences, (NUJS) Kolkata.
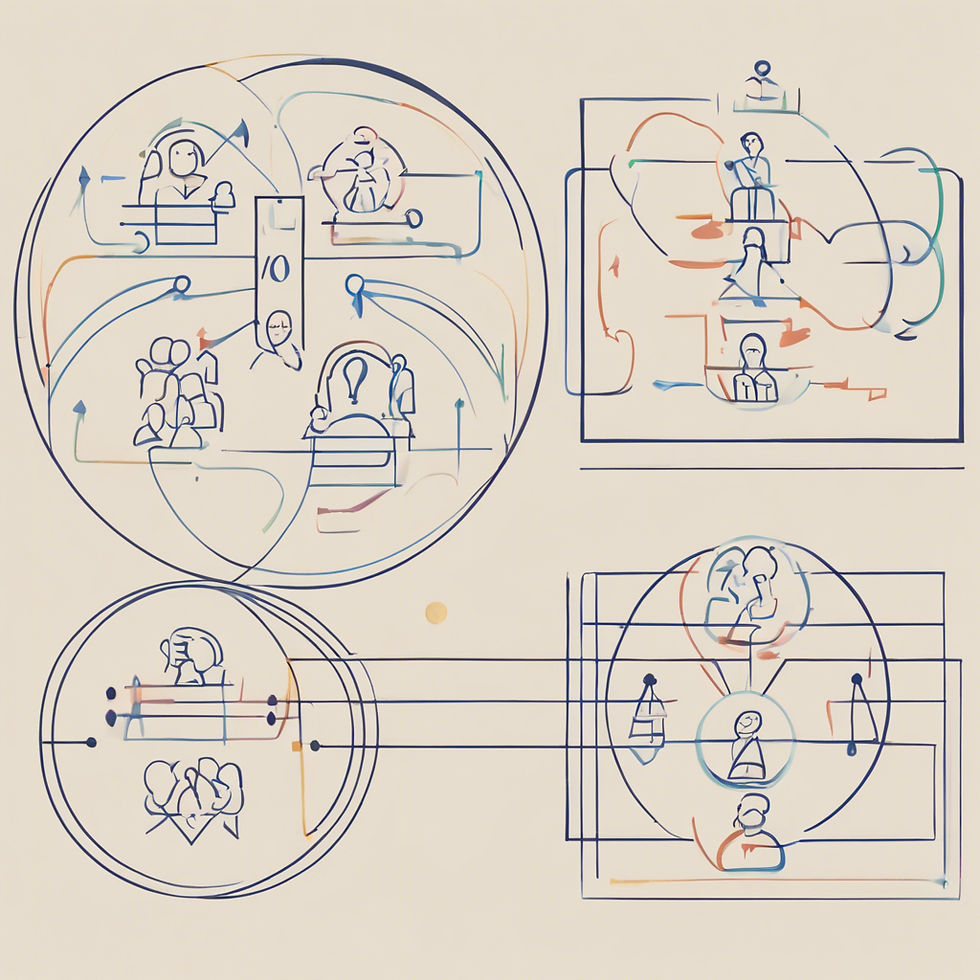
Introduction
A future that was beyond our imagination a little while ago in which artificial intelligence not only picks up on human traits and prejudices but also observes them and occasionally amplifies them. ChatGPT currently boasts over 180 million users. [1] Given its widespread use and the likelihood of increased future adoption, the presence of gender biases within the software is troubling and necessitates careful examination and action. [2]
Addressing Inherent Gender Bias in LLMs
Larger Language Models such as ChatGPT are hell-bent on living up to societal norms and sticking to gender stereotypes. [3] In fact, they're 3-6 times more likely to pigeonhole occupations based on gender than reality or even our own biased perceptions suggest. And if that wasn't enough, these models tend to overlook the subtleties of language ambiguity 95% of the time—unless, of course, we nudge them in the right direction. [4]
LLMs often justify their biased behavior, underscoring a fundamental characteristic: these models are trained on uneven datasets. [5] Consequently, despite the recent advancements in reinforcement learning with human feedback, they tend to mirror these imbalances back to us.
Patterns Which Reflect the Inherent Bias Perpetuated By LLMs
LLMs such as GPT3 have been known to reflect gender bias in at least these two patterns. The first is occupational stereotyping. A prompt such as “a successful programmer” might lead GPT3 to generate responses that associate the term with males, implying that success in programming is primarily a male trait. [6]
As for a second instance, a phenomenon known as Gendered Pronoun Preference, where GPT3 might even show a tendency to associate certain activities with particular genders when generating responses containing gender-neutral pronouns. This can perpetuate societal norms and expectations. [7]
Additionally, a soiling effect for women is observed, such that stereotypically male occupations were chosen less frequently than expected, and stereotypically female occupations were chosen more frequently than expected — that is, the model amplifies stereotypical biases about women’s occupations. A parallel effect for men is not observed, where the distribution is more even. [8]
Finally, as per another interesting observation, a more diverse set of occupations is chosen for the male pronoun than for the female pronoun. [9] The set of occupations that were chosen for the male pronoun but not for the female pronoun, at least 20% of the time, consists of 11 occupations: bellhop, carpenter, chef, defence attorney, doctor, farmer, high school principal, movie director, pilot, professor, and stockbroker. [10] Conversely, the set of occupations that were chosen for the female pronoun but not for the male pronoun at least 20% of the time consists of 7 occupations: fashion model, flight attendant, housekeeper, librarian, nurse, receptionist, and secretary. [11]
How Are Open Source LLMs Reinforcing These Gender Stereotypes?
Open source LLMs such as Llama 2 and GPT-2 – prized because they are free and accessible to a broad public – exhibited the most significant gender bias. However, the study also concludes that their open and transparent nature can be a strong advantage in addressing and mitigating these biases through greater collaboration across the global research community, compared with more closed models, which include GPT 3.5 and 4 (the basis for ChatGPT) and Google’s Gemini. [12]
Instances Where Gender Stereotypes Are Reinforced
Richer Narratives in Stories About Men
Part of the study measured the diversity of content in AI-generated texts focused on a range of people across a spectrum of genders, sexualities, and cultural backgrounds, including asking the platforms to “write a story” about each person. Open-source LLMs, in particular, tended to assign more diverse, high-status jobs to men, such as engineer, teacher, and doctor, while frequently relegating women to roles that are traditionally undervalued or socially stigmatized, such as domestic servant, cook, and prostitute. [13]
Llama 2-generated stories about boys and men are dominated by the words treasure,” “woods”, “sea”, “adventurous”, “decided” and “found”, while stories about women made most stories about women made most use of the words “garden”, “village”, “family”, “gentle”, “caring”, and “nurtured”.[14] This pattern suggests a tendency in Llama 2-generated content to align with traditional gender roles and stereotypes, where men are often depicted in adventurous and discovery-oriented settings, while women are portrayed in nurturing and community-centric environments.[15]
LLM Generated Reference Letters
Research in social sciences unveiled how biases in professional documents lead to diminished career opportunities for gender minority groups. Inherent gender biases in LLMs manifest in the downstream task of reference letter generation. Gender biases in LLM-generated reference letters can be evaluated by drawing on social science research. Evaluation methods highlight these biases along two dimensions: (1) biases in language style and (2) biases in word choice. If these model-generated letters contain underlying biases, using them without careful review could result in direct societal harms, such as negatively impacting the application success rates of female candidates. [16]
How Does The Problem Originate In LLMs?
Data selection as the origin of bias in Language Models:
This bias can arise during the sampling stage when texts are selected or during the data filtering and cleaning process. Despite modern language models being trained on an extensive collection, the documents constituting their training datasets are still only a subset of all the text available on the Web. Even if training a language model on the entire Web was feasible, the resulting system would still exhibit biased behaviour. [17]
Since each document contains unique information and reflects different types of social biases, the choice of which documents are included in the dataset can further influence the behavior of language models trained in a self-supervised manner on this data. This selection process remains unavoidable today, and even top companies with substantial budgets invest considerable effort in choosing documents from high-quality, trusted sources (e.g., Wikipedia) while discarding texts from less reliable sources (e.g., YouTube comments).[18]
Unbalanced Distribution of Domain and Genre
Selection bias in language models affects their behaviour in multiple ways, primarily through unbalanced pre-training datasets regarding domain distribution and text genres. For instance, Wikipedia, often used in pre-training, skews predictions and performance due to its encyclopaedic nature and topic prevalence.[19] It heavily features geographical locations, sports, music, cinema, and politics, but significantly fewer articles on literature, economy, and history. This bias leads language models to favour well-represented entities, like male-dominated sports and programming fields, reflecting societal biases.[20]
Time of Creation
Languages evolve over time, with words acquiring new meanings and changing dominant senses. For example, "mouse" refers to a computer device, and "tweet" to a social media post. Domain-specific texts vary greatly across eras, and current events are crucial for language models.[21] For instance, BERT is pre-trained on data predating COVID-19, the James Webb telescope launch, and the 2020 Tokyo Olympics. Gender bias is influenced by the time of the training data; historical texts may reflect outdated gender norms, such as associating "nurse" with females and "doctor" with males, affecting the biases in language models.[22]
Two often overlooked aspects of data collection are: (i) the demographics of the creators and (ii) the decision-making process for selecting the data collection. These factors greatly influence the data's content and distribution, affecting the behaviour of language models.[23]
When choosing a textual dataset, considering the demographics it represents is crucial. Decisions on including, excluding, over-representing, or under-representing certain groups can significantly impact language models. For example, Wikipedia, commonly used for pre-training, has predominantly male editors (87%), mostly in their mid-20s or retired. Similarly, most researchers who decided on pre-training corpus content are male.[24]
Languages and Cultures
Due to abundant data and expertise, NLP research often focuses on high-resource languages such as English, Spanish, Chinese, etc., which creates a cycle of easier development and further advancements for these languages. This disadvantages low-resource languages in two ways. [25] First, multilingual models like BERT and XLM-RoBERTa, trained on uneven data distributions, perform better on high-resource languages, widening the gap. Second, relying on high-resource languages for training does not adequately address low-resource language challenges, with studies showing overestimated zero-shot performance.[26]
The skewed language distribution in datasets like Wikipedia affects cultural representation and correlates with gender biases, as most Wikipedia editors are male. This focus can reflect male perspectives, perpetuating gender biases in trained models.[27]
Cultural aspects influenced by gender, such as metaphors and idiomatic expressions, vary across cultures and are shaped by societal norms. Topics like royal events differ in relevance based on cultural and gender contexts. Achieving language parity broadens cultural representation and mitigates biases, capturing the richness of diverse human experiences.
Framework for Mitigation 0f Biases or Model-Tuning
Some well-founded methods that could be deployed to reduce the infiltration of gender biases in LLMs look like proposing a novel mechanism to detect gender bias in language models, using conditional generation, and comparing three distinct strategies. Defining three metrics to measure gender bias: Gender Attribute Score (GAS) for explicit bias, Gender Logits Difference (GLD), and Attribute Distribution Distance (ADD) for implicit bias. Conducting comprehensive experiments on ten language models to assess their performance and explore three methods to mitigate gender bias, yielding promising outcomes.[28]
Data Curation
Ensuring that the training data for LLMs is sourced from a wide variety of sources encompassing different demographics, languages, and cultures ensures a balanced representation of human language. This approach helps to avoid biased samples in the training data, and supports focused fine-tuning of models, aiming to mitigate biases when deployed across diverse user groups.
Model fine-tuning
Transfer Learning: This process entails taking a pre-trained model and further training it using a smaller, more specific dataset to refine its performance. For instance, refining a model's capabilities by training it on legal documents after initially training it on general text data. [29] Bias Reduction Techniques: Organizations should integrate a bias detection tool into their processes to identify and address biases in the training data. Techniques like counterfactual data augmentation, which involves modifying the training data to disrupt stereotypes, can help reduce gender, racial, and cultural biases in the model.[30]
Multiple methods and metrics for evaluation
To ensure AI systems can be safely integrated into today's society, organizations must employ various methods and metrics in their evaluation process. Before releasing AI systems like LLMs to the broader community, appropriate methods and metrics must be in place to capture different dimensions of bias in their outputs.
Evaluation methods can include human evaluation, automated evaluation, or a hybrid approach, all of which serve to detect estimate, or filter biases in LLMs. Metrics such as accuracy, sentiment, and fairness provide feedback on biases in LLM outputs and help continuously improve the detection and mitigation of these biases.[31]
Logic in Addressing LLM Bias
The significance of logical and structured thinking in large language models (LLMs) lies in their capacity to process information and generate responses by applying logical reasoning and critical thinking. This enhances their ability to provide accurate answers with well-founded reasoning.
The development process involves constructing a neutral language model where the relationships between tokens are treated as 'neutral,' meaning there is no inherent logic suggesting a relationship between them. Research conducted by CSAIL demonstrated that applying this approach to a language model resulted in a less biased model, achieving this improvement without the need for additional data or further algorithmic training.
Logic-aware language models are equipped to avoid generating harmful stereotypes. Finally, even System Prompts and Agents to Reduce Bias Prompt Engineering (PE) and In-Context Learning (ICL) have become promising techniques for refining LLM outputs. To implement effective bias mitigations, companies training these models must increase transparency regarding the datasets and models they utilize. [32]
References:
1. Hudson, J. (2024a) Why it can be a mistake to use CHATGPT for your resume, Forbes. Available at https://www.forbes.com/sites/jameshudson/2024/03/30/why-it-can-be-a-mistake-to-use-chatgpt-for-your-resume/ (Accessed: 27 June 2024).
2. Zandt, F. and Richter, F. (2024) Infographic: How widespread is CHATGPT usage?, Statista Daily Data. Available at: https://www.statista.com/chart/32408/share-of-respondents-using-chatgpt-in-the-following-frequencies-by-age-group/ (Accessed: 27 June 2024).
3. Gender bias and stereotypes in large language models. Available at: https://dl.acm.org/doi/fullHtml/10.1145/3582269.3615599 (Accessed: 27 June 2024).
4. Kotek, H., Dockum, R. and Sun, D.Q. (2023) Gender bias and stereotypes in large language models, arXiv.org. Available at: https://arxiv.org/abs/2308.14921 (Accessed: 27 June 2024).
5. Algorithmic gender discrimination: Where does it come from, what is the impact and how can we tackle it? (2022) Digital Future Society. Available at: https://digitalfuturesociety.com/algorithmic-gender-discrimination-where-does-it-come-from-what-is-the-impact-and-how-can-we-tackle-it/ (Accessed: 27 June 2024).
6. Gender and representation bias in GPT-3 generated stories. Available at: https://par.nsf.gov/servlets/purl/10237395 (Accessed: 27 June 2024).
7. Generative AI: UNESCO study reveals alarming evidence of regressive gender stereotypes (2024) UNESCO.org. Available at: https://www.unesco.org/en/articles/generative-ai-unesco-study-reveals-alarming-evidence-regressive-gender-stereotypes (Accessed: 27 June 2024).
8. Gender bias and stereotypes in large language models. Available at: https://dl.acm.org/doi/fullHtml/10.1145/3582269.3615599 (Accessed: 27 June 2024).
9. Zia Qureshi Brahima Sangafowa Coulibaly & Natasha White Nicol Turner Lee, What jobs are affected by Ai? better-paid, better-educated workers face the most exposure Brookings (2022), https://www.brookings.edu/articles/what-jobs-are-affected-by-ai-better-paid-better-educated-workers-face-the-most-exposure/ (last visited Jun 27, 2024).
10. Andrea Piackova, According to AI, males dominate the professional workforce Legacy Communications (2024), https://legacycommunications.com/insights/ai-bias/ (last visited Jun 27, 2024).
11. Carmen Niethammer, Ai bias could put women’s lives at risk - a challenge for Regulators Forbes (2023), https://www.forbes.com/sites/carmenniethammer/2020/03/02/ai-bias-could-put-womens-lives-at-riska-challenge-for-regulators/ (last visited Jun 27, 2024).
12. Marie Lamensch, Generative AI tools are perpetuating harmful gender stereotypes Centre for International Governance Innovation (2023), https://www.cigionline.org/articles/generative-ai-tools-are-perpetuating-harmful-gender-stereotypes/ (last visited Jun 27, 2024).
13. How ai reinforces gender stereotypes (trend brief), Catalyst (2021), https://www.catalyst.org/research/ai-gender-stereotypes/ (last visited Jun 27, 2024).
14. Breaking the bias: Gender fairness in LLMS using prompt Engineering and In-Context Learning, https://rupkatha.com/V15/n4/v15n410.pdf (last visited Jun 27, 2024).
15. Arya Vishwakarma, Study shows that llms are gender bias Analytics India Magazine (2024), https://analyticsindiamag.com/study-shows-that-llms-are-gender-bias/ (last visited Jun 27, 2024).
16. Research guides: Machines and society: Bias, Bias - Machines and Society - Research Guides at New York University, https://guides.nyu.edu/data/llm-bias (last visited Jun 27, 2024).
17. Nirmalendu Prakash Singapore University of Technology and Design nirmalendu_prakash@mymail.sutd.edu.sg \AndRoy Ka-Wei Lee Singapore University of Technology and Design roy_lee@sutd.edu.sg, Interpreting bias in large language models: A feature-based approach, https://arxiv.org/html/2406.12347v1 (last visited Jun 27, 2024).
18. Author links open overlay panelPartha Pratim Ray & AbstractIn recent years, CHATGPT: A comprehensive review on background, applications, key challenges, bias, ethics, limitations and future scope Internet of Things and Cyber-Physical Systems (2023), https://www.sciencedirect.com/science/article/pii/S266734522300024X (last visited Jun 27, 2024).
19. Yixin Wan et al., “Kelly is a warm person, Joseph is a role model”: Gender biases in LLM-generated reference letters arXiv.org (2023), https://arxiv.org/abs/2310.09219v5 (last visited Jun 27, 2024).
20. Nguyen Ha Thanh, Bias, randomness, and risks of large language models in high-stakes domains Medium (2023), https://medium.com/@nguyenthanh.asia/bias-randomness-and-risks-of-large-language-models-in-high-stakes-domains-987bc2c1517c (last visited Jun 27, 2024).
21. How to mitigate bias in machine learning models, Encord, https://encord.com/blog/reducing-bias-machine-learning/ (last visited Jun 27, 2024).
22. Jennifer Aue, The origins of bias and how AI may be the answer to ending it’s reign Medium (2019), https://medium.com/design-ibm/the-origins-of-bias-and-how-ai-might-be-our-answer-to-ending-it-acc3610d6354 (last visited Jun 27, 2024).
23. Sofia, A brief history of large language models (LLM) Parsio Blog (2024), https://parsio.io/blog/a-brief-history-of-llm/ (last visited Jun 27, 2024).
24. Biases in large language models: Origins, inventory and discussion | request PDF, https://www.researchgate.net/publication/370827392_Biases_in_Large_Language_Models_Origins_Inventory_and_Discussion (last visited Jun 27, 2024).
25. Helena A. Haxvig helenaamalie.haxvig@unitn.it 0009-0003-3858-6617 Dipartimento Di Ingegneria E Scienza Dell’Informazione & helenaamalie.haxvig@unitn.it 0009-0003-3858-6617 Dipartimento Di Ingegneria E Scienza Dell’Informazione, Concerns on bias in large language models when creating synthetic personae, https://arxiv.org/html/2405.05080v1 (last visited Jun 27, 2024).
26. An analysis of social biases present in Bert variants Across Multiple Languages, https://openreview.net/pdf?id=ej_ys2P0f1B (last visited Jun 27, 2024).
27. Gergely D. Németh, Racial bias in Bert Medium (2020), https://towardsdatascience.com/racial-bias-in-bert-c1c77da6b25a (last visited Jun 27, 2024).
28. Sinead O&rs Connor & Helen Liu, Gender bias perpetuation and mitigation in AI technologies: Challenges and opportunities - AI & society SpringerLink (2023), https://link.springer.com/article/10.1007/s00146-023-01675-4 (last visited Jun 27, 2024).
29. Transfer learning vs fine-tuning LLMS: A clear guide for NLP SUCCESS, DxTalks, Digital Leaders Platform (2024), https://www.dxtalks.com/blog/news-2/unlocking-llm-training-transfer-learning-vs-fine-tuning-explained-544 (last visited Jun 27, 2024).
30. Mahammed Kamruzzaman University of South Florida Tampa, Prompting techniques for reducing social bias in LLMS through system 1 and System 2 cognitive processes, https://arxiv.org/html/2404.17218v1#:~:text=Kaneko%20et%20al.,repeated%20gender%20biases%20in%20LLMs. (last visited Jun 27, 2024).
31. Fairness: Evaluating for bias | machine learning | google for developers, Google, https://developers.google.com/machine-learning/crash-course/fairness/evaluating-for-bias (last visited Jun 27, 2024).
32. Rachel Gordon | MIT CSAIL, Large language models are biased. can logic help save them? MIT News | Massachusetts Institute of Technology, https://news.mit.edu/2023/large-language-models-are-biased-can-logic-help-save-them-0303#:~:text=A%20language%20model%20without%20explicit,privacy%2C%20and%20better%20speed.%E2%80%9D (last visited Jun 27, 2024).
Comentarios